Hi @skr3178!
The other bands have different values as the highest in their category.
Some at 10500, others 11000, etc for example.
Yes, you are correct, the band values can end up larger than 10000, because a pixel can be affected by the reflectance of neighboring pixels, so this is not unusual and can happen also to the RGB bands.
In reality, these values are ignored via cutoff, which I would suggest you do as well. For example, when I usually plot images with matplotlib
in python, I do the following if I work with DN values:
import numpy as np
import matplotlib.pyplot as plt
# data is of shape (13, 40, 40)
# reshape data
ndata = np.moveaxis(data, 0, -1) # ndata is of shape (40, 40, 13)
# select RGB (or any other combination)
rgb = ndata[..., [4,3,2]]
# plot image
plt.imshow(rgb/10000*3.5)
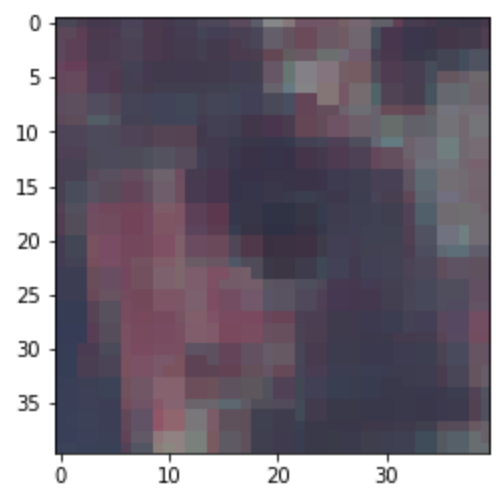
matplotlib
draws float
values from [0.0, 1.0]
and maps them to [0, 255]
when drawing an image (out of range values are ignored), so this is why I divided with 10000 to get the reflectances, and then multiplied with 3.5 to increase the range so that the image is brighter (generally the values are at 0.4 and below).
The same can be achieved with the following cutoff procedure and plotting 8bit integer data directly:
ndata_cutoff = np.clip(ndata/10000, 0, 0.3) # divide with 10000 and cut of to range [0.0, 0.3]
ndata_normalized = ndata_cutoff/0.3 # stretch to [0.0, 1.0]
ndata_8bit = (ndata_normalized*255).astype(np.uint8) # stretch to 255 and convert to uint8
false_color = ndata_8bit[..., [8,4,3]] # select false color bands for plotting
plt.imshow(false_color)
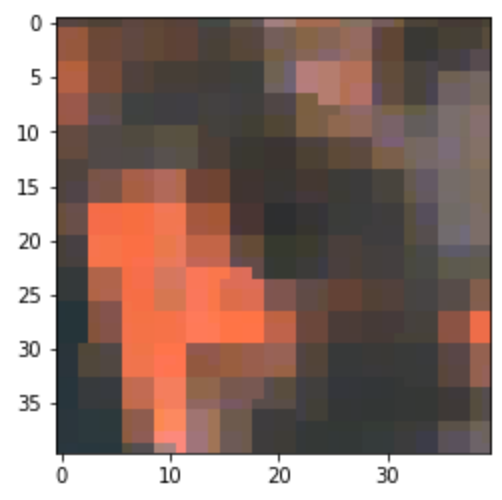
I would say the normalization procedure shown is good for any kind of band, exceptions are weird locations, like bright deserts, clouds, etc.
Hope this helps! Let me know if there’s anything still unclear.
Cheers,
Matic